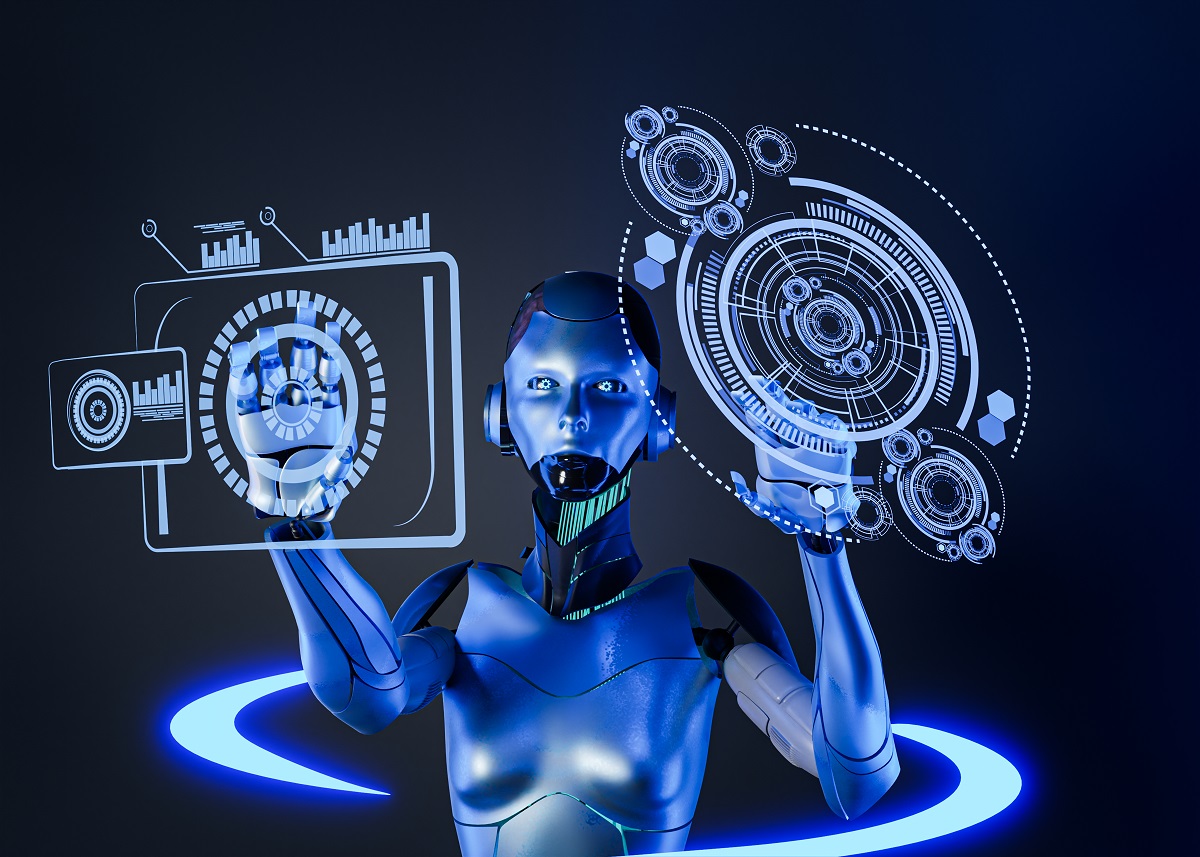
Generative AI in Testing Market By Testing Types (Functional Testing, Performance Testing, Security Testing, User Interface Testing, and Others), By Industry, By Deployment Mode (On-premises and Cloud-based), By Applications, By Region and Companies - Industry Segment Outlook, Market Assessment, Competition Scenario, Trends, and Forecast 2023-2032
-
38032
-
July 2023
-
179
-
-
This report was compiled by Correspondence Linkedin | Detailed Market research Methodology Our methodology involves a mix of primary research, including interviews with leading mental health experts, and secondary research from reputable medical journals and databases. View Detailed Methodology Page
-
Report Overview
Generative AI in Testing Market size is expected to be worth around USD 150.4 Mn by 2032 from USD 32 Mn in 2022, growing at a CAGR of 17.2% during the forecast period from 2023 to 2032.
The global generative AI in testing market refers to the market for using generative artificial intelligence (AI) techniques and algorithms to enhance and automate the software testing process. Generative AI in testing involves the use of AI models to generate test cases, data, and scenarios, enabling efficient and comprehensive testing of software applications.
Generative AI in testing offers several advantages over traditional manual testing methods. It can significantly speed up the testing process by automatically generating a large number of test cases and data that cover various scenarios and edge cases. This helps improve test coverage and ensures the thorough validation of software applications. Additionally, generative AI in testing can identify patterns, trends, & anomalies in data, enabling more effective detection of bugs, vulnerabilities, and performance issues.
Market Scope
Testing Types Analysis
Based on the testing types, the market for generative AI in testing is segmented into functional testing, performance testing, security testing, user interface testing, and other testing types. Among that functional testing segment is dominant in the market with a market share of 32%.
Generative AI can be used to generate test cases by learning patterns and behaviors from existing functional test data. By training on a dataset of valid and invalid inputs, generative AI models can generate new test cases that cover different scenarios and increase test coverage.
Generative AI can play a role in performance testing by generating realistic workloads and simulating user behavior patterns. Generative AI techniques can aid in security testing by generating malicious inputs or attack scenarios. By training on known vulnerabilities or attack patterns, generative AI models can generate synthetic malicious inputs to test an application or system's security measures and defenses.
Generative AI can also be applied to other testing types, such as compatibility testing, accessibility testing, and localization testing. By training on relevant datasets and specifications, generative AI models can generate test cases that cover different device configurations, accessibility requirements, and language variations.
Industry Analysis
By industry, the market for generative AI in testing is segmented into IT and telecommunications, finance, retail, automotive, healthcare, and other industries. From that IT and telecommunications industry is dominant in the market, with a market share of about 29%.
In the IT and telecommunications industry, generative AI can be utilized to automate the generation of test cases and simulate various scenarios to identify bugs and vulnerabilities in software applications, network infrastructure, and communication systems.
In finance, generative AI can aid in testing financial software, algorithms, and trading systems. It can generate synthetic data sets to mimic real-world market conditions and identify potential risks and anomalies. Generative AI in Testing Market can have significant applications in the healthcare industry. It can be used to test medical devices, software applications, and algorithms used in diagnostics, treatment planning, and patient monitoring.
Generative AI in testing is applicable across various other industries as well. For example, in manufacturing, it can be used to optimize production processes, test product designs, and identify potential quality issues. In energy and utilities, generative AI can aid in testing smart grid systems, optimizing energy distribution, and predicting equipment failures.
Deployment Mode Analysis
Based on the deployment mode, the market is segmented into on-premises and cloud-based. In an on-premises deployment mode, the generative AI in testing solution is installed and hosted within the organization's infrastructure. This means the software is deployed and operated on the company's servers and data centers, typically located within their premises.
On-premises deployment offers organizations greater control and customization options over the solution, as it is managed internally. Cloud-based deployment mode involves hosting the generative AI in testing solutions on remote servers provided by a third-party cloud service provider. With this model, organizations do not need to invest in their own hardware infrastructure nor maintain and update it themselves. Cloud-based deployment offers scalability, flexibility, and easier access to updates and new features.
Applications Analysis
Based on the application, the Generative AI in Testing Market is segmented into test case generation, bug detection and diagnosis, test data generation, test environment simulation, and intelligent test prioritization. Generative AI can automate the process of generating test cases by analyzing the software under test and its requirements. It can create a wide range of test scenarios, inputs, and conditions to ensure thorough testing coverage.
Generative AI can assist in the detection & diagnosis of bugs and issues in software applications. By analyzing vast amounts of data, including code, logs, and execution traces, generative AI algorithms can identify patterns, anomalies, and potential areas of concern. Generative AI can generate synthetic test data that closely resembles real-world data to facilitate testing. It can generate diverse datasets with varying characteristics, ensuring comprehensive coverage of different data scenarios.
Generative AI can simulate complex test environments by emulating different systems, networks, & configurations. It can create virtualized environments that closely mimic real-world conditions, allowing for more accurate and realistic testing. Generative AI can analyze various factors, such as code changes, bug history, test coverage, and business priorities, to intelligently prioritize test cases. It can identify critical test cases that are more likely to uncover issues or impact the software more.
Key Market Segments
Based on Testing Types
- Functional Testing
- Performance Testing
- Security Testing
- User Interface Testing
- Other Testing Types
Based on Industry
- IT and Telecommunications
- Finance
- Retail
- Automotive
- Healthcare
- Other Industries
Based on the Deployment Mode
- On-premises
- Cloud-based
Based on Applications
- Test Case Generation
- Bug Detection and Diagnosis
- Test Data Generation
- Test Environment Simulation
- Intelligent Test Prioritization
Drivers
Multiple key factors drive the global generative AI in testing market. Chief among them is increasing software complexity and demand, coupled with efficient testing that meets that need, prompting the adoption of this form of artificial intelligence in testing. As technology rapidly progresses and demands grow for sophisticated software systems, manual testing methods often cannot keep pace with them; Generative AI provides organizations with a solution by automating and augmenting testing procedures allowing greater test coverage as well as more precise validation.
Secondly, the rising adoption of Agile and DevOps methodologies is fueling the demand for generative AI in testing. Agile and DevOps emphasize continuous integration, frequent releases, and shorter development cycles. These approaches require robust and efficient testing practices to ensure software quality and rapid deployment. Generative AI in testing aligns well with Agile and DevOps workflows by enabling automated and continuous testing throughout the software development lifecycle. It helps organizations accelerate their testing cycles, provide faster feedback, and deliver high-quality software at a faster pace.
Furthermore, the advancements in AI and machine learning technologies play a significant role in driving generative AI in testing market. AI models are becoming more sophisticated in analyzing complex data, simulating user interactions, and generating realistic test scenarios. With large-scale datasets and improved computational capabilities, AI models can be trained specifically for testing purposes. These advancements enable organizations to leverage generative AI in testing to achieve higher accuracy, efficiency, and reliability in their software testing efforts.
Restraints
The global generative AI in testing market also faces certain restraints that can impact its growth and adoption. Firstly, the lack of understanding and expertise in implementing and utilizing generative AI in testing can be a significant restraint. Integrating generative AI techniques into existing testing processes requires a certain level of knowledge and expertise in AI and machine learning. Organizations may face challenges in acquiring the necessary skills, training their personnel, or hiring qualified professionals in this specialized field. The complexity of AI models and algorithms used in generative AI in testing can further contribute to this restraint.
Secondly, concerns about generative AI's reliability and accuracy in testing may impede its adoption. While generative AI techniques have shown promising results, there is always a level of uncertainty associated with AI-generated test cases and scenarios. Organizations may be cautious about fully relying on generative AI in testing, especially for critical or high-risk applications, as the potential for false positives or false negatives in test results could have significant consequences. Building trust and ensuring the robustness and reliability of generative AI models are crucial factors in addressing this restraint.
Another restraint is the potential ethical and legal considerations associated with generative AI in testing. AI-generated test cases and scenarios must comply with legal and ethical standards, including data privacy regulations and intellectual property rights. Ensuring that generative AI models do not infringe upon user privacy, disclose sensitive information, or violate any legal restrictions is crucial. Organizations need to carefully navigate these ethical and legal considerations to avoid potential liabilities or reputational damage.
Opportunity
The global generative AI in testing market presents several opportunities that can drive its growth and expansion. Firstly, the need for efficient and comprehensive testing solutions is growing as software applications become increasingly complex and interconnected. Generative AI in testing offers the opportunity to automate and augment the testing process, enabling organizations to achieve higher test coverage, identify critical bugs, and improve software quality. The ability of generative AI to generate a large number of test cases and scenarios, including edge cases and corner cases, presents an opportunity to enhance the effectiveness and efficiency of software testing.
Second, the increase in Agile and DevOps adoption requires organizations to integrate testing into continuous integration and delivery pipelines. Generative AI testing offers organizations an ideal solution, seamlessly blending into these methodologies for automated and continuous testing throughout the software development lifecycle - thus streamlining testing processes, speeding time-to-market, and improving quality. This partnership presents organizations with ample opportunity to streamline testing procedures while speeding up time-to-market.
Advancements in AI and machine learning technologies create new opportunities for expanding generative AI testing. AI models are becoming more sophisticated at analyzing data, simulating user behavior, detecting patterns and anomalies, and finding patterns and anomalies - these advancements present an opportunity to develop further generative AI capabilities and accuracy, resulting in increased test coverage, bug detection, and overall software quality enhancements.
Moreover, the increasing focus on security and privacy in software applications creates opportunities for generative AI in testing. Security vulnerabilities and privacy breaches pose significant risks to organizations and their customers. Generative AI in testing can help identify security vulnerabilities, simulate attacks, and uncover potential privacy issues by generating test scenarios and data that cover a wide range of security and privacy aspects. This presents an opportunity for organizations to leverage generative AI in testing to strengthen the security and privacy of their software applications.
Trends
The global generative AI in testing market is witnessing several trends that are shaping its trajectory. Firstly, there is a growing trend towards the integration of generative AI in testing into the overall software development lifecycle. Organizations are recognizing the benefits of incorporating generative AI techniques early in the development process to identify and address issues at an early stage. By integrating generative AI in testing, organizations can achieve faster feedback loops, reduce development costs, and deliver higher-quality software products.
Secondly, explainable AI (XAI) is emerging as a significant trend in the generative AI in testing market. As AI models generate test cases and scenarios, it becomes crucial to understand the reasoning behind their decisions. Explainable AI enables developers and testers to understand why a specific test case was generated or why a particular scenario was chosen, providing transparency and insights into the testing process. This trend helps build trust in generative AI in testing and facilitates collaboration between AI models and human testers.
Another notable trend is the focus on diversity and inclusivity in generative AI in testing. AI models should be trained on diverse datasets representing user demographics, cultural backgrounds, and usage patterns. This ensures that the generated test cases and scenarios cover a wide range of real-world situations and accurately reflect the user base. The trend towards diversity and inclusivity promotes fairness and reduces biases in generative AI in testing, leading to more reliable and comprehensive testing results.
Regional Analysis
North America, and particularly the United States, is often at the forefront of technological developments. The region boasts an established software testing industry and an emphasis on innovation. Major technology hubs such as Silicon Valley encourage the adoption of generative AI testing through research and development investments by companies.
North America currently holds a 38% market share. Europe boasts more mature software development industries with well-established quality assurance practices in place; Germany, the United Kingdom, and France all boast advanced testing practices and regulations that guarantee quality assurance standards are upheld across their borders.
The Asia-Pacific region has experienced a considerable expansion in the software industry over recent years. Countries like China, India, and Japan are well known for their technological developments; as these nations increasingly adopt automation and AI technologies, generative AI testing applications should increase. Furthermore, emerging economies in Southeast Asia present opportunities to further extend this adoption.
Latin America boasts a flourishing software industry, with countries like Brazil, Mexico, and Argentina playing key roles. While the adoption of generative AI testing is slower in Latin America than in other regions, its adoption will gain ground as companies strive to optimize testing efficiency while simultaneously improving product quality.
The Middle East and Africa regions are experiencing increased digital transformation initiatives, driving the expansion of the software industry. As organizations invest in technology infrastructure and software development projects, the adoption of generative AI testing techniques could prove useful in increasing effectiveness and speed during testing processes.
Key Regions
- North America
- The US
- Canada
- Mexico
- Western Europe
- Germany
- France
- The UK
- Spain
- Italy
- Portugal
- Ireland
- Austria
- Switzerland
- Benelux
- Nordic
- Rest of Western Europe
- Eastern Europe
- Russia
- Poland
- The Czech Republic
- Greece
- Rest of Eastern Europe
- APAC
- China
- Japan
- South Korea
- India
- Australia & New Zealand
- Indonesia
- Malaysia
- Philippines
- Singapore
- Thailand
- Vietnam
- Rest of APAC
- Latin America
- Brazil
- Colombia
- Chile
- Argentina
- Costa Rica
- Rest of Latin America
- Middle East & Africa
- Algeria
- Egypt
- Israel
- Kuwait
- Nigeria
- Saudi Arabia
- South Africa
- Turkey
- United Arab Emirates
- Rest of MEA
Key Players Analysis
The generative AI in testing market was still emerging, and specific market share data might be limited. However, there are several key players that have made significant contributions and advancements in this space. Some of the prominent companies operating in the generative AI in testing market include:
OpenAI: OpenAI is a leading organization in artificial intelligence, and they have developed advanced generative models such as GPT-3. While their primary focus is on natural language processing, their models have potential applications in various domains, including testing.
Appvance.ai: Appvance.ai offers a comprehensive AI-driven testing platform that utilizes generative AI techniques to generate test data and test cases. Their platform enables organizations to automate and enhance their testing processes through AI-driven test creation and execution.
Listed below are some of the most prominent generative AI in testing industry players.
Market Key Players
- Applitools
- Functionize
- Mabl
- Diffblue
- AdaLogica
- Appvance
- ai
- Other Key Players
Recent Developments
- In 2021: ai: Appvance.ai introduced advancements in their AI-driven testing platform, including enhancements to their generative AI algorithms for test data generation and automated test case creation. These developments aimed to improve the efficiency and effectiveness of testing processes.
- In 2020: Functionize: Functionize focused on expanding the capabilities of their AI-powered testing platform, leveraging generative AI techniques. They introduced features such as intelligent test maintenance and self-healing tests, which utilize machine-learning algorithms to adapt to changes in the application and optimize test execution.
Report Scope
Report Features Description Market Value (2022) USD 32 Mn Forecast Revenue (2032) USD 150.4 Mn CAGR (2023-2032) 17.2% Base Year for Estimation 2022 Historic Period 2016-2022 Forecast Period 2023-2032 Report Coverage Revenue Forecast, Market Dynamics, COVID-19 Impact, Competitive Landscape, Recent Developments Segments Covered By Testing Types (Functional Testing, Performance Testing, Security Testing, User Interface Testing, and Other Testing Types) By Industry (IT and Telecommunications, Finance, Retail, Automotive, Healthcare, and Other Industries)
By Deployment Mode (On-premises and Cloud-based)
By Applications (Test Case Generation, Bug Detection, and Diagnosis, Test Data Generation, Test Environment Simulation, and Intelligent Test Prioritization)Regional Analysis North America – The US, Canada, & Mexico; Western Europe – Germany, France, The UK, Spain, Italy, Portugal, Ireland, Austria, Switzerland, Benelux, Nordic, & Rest of Western Europe; Eastern Europe – Russia, Poland, The Czech Republic, Greece, & Rest of Eastern Europe; APAC – China, Japan, South Korea, India, Australia & New Zealand, Indonesia, Malaysia, Philippines, Singapore, Thailand, Vietnam, & Rest of APAC; Latin America – Brazil, Colombia, Chile, Argentina, Costa Rica, & Rest of Latin America; the Middle East & Africa – Algeria, Egypt, Israel, Kuwait, Nigeria, Saudi Arabia, South Africa, Turkey, United Arab Emirates, & Rest of MEA Competitive Landscape Applitools, Functionize, Mabl, Diffblue, AdaLogica, Appvance, Morph.ai, and Other Key Players Customization Scope Customization for segments, region/country-level will be provided. Moreover, additional customization can be done based on the requirements. Purchase Options We have three licenses to opt for: Single User License, Multi-User License (Up to 5 Users), Corporate Use License (Unlimited User and Printable PDF) -
-
- Applitools
- Functionize
- Mabl
- Diffblue
- AdaLogica
- Appvance
- ai
- Other Key Players