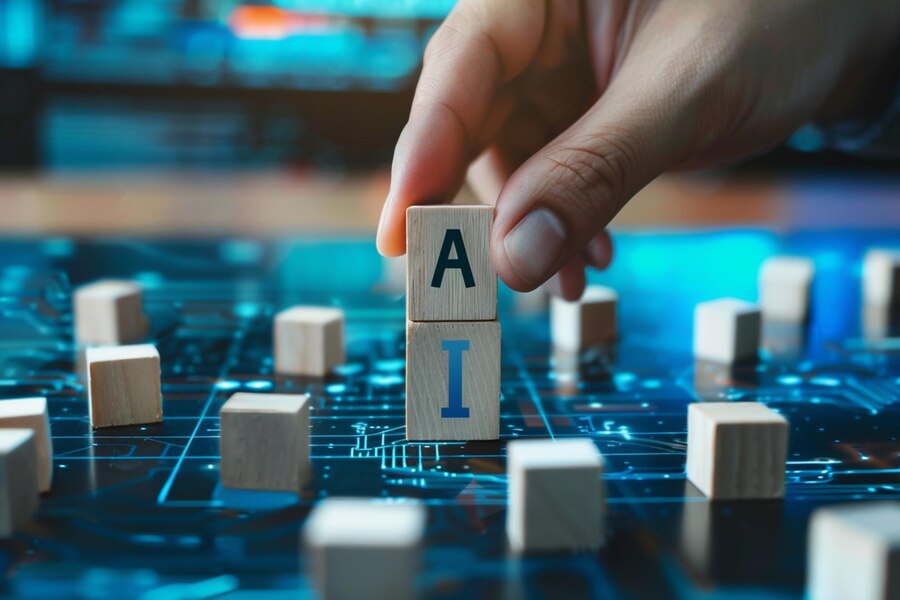
Generative AI in Data Labeling Solution and Services Market By Sourcing Type (In-House, Outsourced), By Type (Audio-Based, Image/Video-Based, Text-Based), By Labeling Type (Automatic, Manual, Semi-Supervised), By Vertical (Automotive, Financial Services, Government, Healthcare, IT Data, Retail, Others), By Region and Companies - Industry Segment Outlook, Market Assessment, Competition Scenario, Trends and Forecast 2024-2033
-
50732
-
Aug 2024
-
300
-
-
This report was compiled by Vishwa Gaul Vishwa is an experienced market research and consulting professional with over 8 years of expertise in the ICT industry, contributing to over 700 reports across telecommunications, software, hardware, and digital solutions. Correspondence Team Lead- ICT Linkedin | Detailed Market research Methodology Our methodology involves a mix of primary research, including interviews with leading mental health experts, and secondary research from reputable medical journals and databases. View Detailed Methodology Page
-
Quick Navigation
Report Overview
The Global Generative AI in Data Labeling Solution and Services Market was valued at USD 11.9 Bn in 2023. It is expected to reach USD 84.0 Bn by 2033, with a CAGR of 22.2% during the forecast period from 2024 to 2033.
The Generative AI in Data Labeling Solution and Services Market focuses on providing advanced tools and services for labeling data to train generative AI models effectively. As AI applications become more sophisticated, particularly in natural language processing and computer vision, the need for accurate, diverse, and extensive data labeling has grown significantly. This market is driven by the demand for high-quality datasets to train large language models like GPT-3 and to minimize biases in AI outputs, making it critical for sectors relying on AI for decision-making and automation.
The Generative AI in Data Labeling Solution and Services Market is poised for significant expansion, driven by the escalating complexity and scale of AI models like GPT-3, which are trained on vast amounts of data—over 570 gigabytes in some cases. As generative AI applications proliferate across industries, the demand for comprehensive and precise data labeling solutions has surged, ensuring that AI models are not only effective but also relevant to their intended use cases.
One of the critical factors driving this market is the need to reduce bias in AI models, particularly in sensitive applications such as hiring, law enforcement, and financial services. Data labeling efforts that prioritize diversity can reduce bias by up to 40%, enhancing the fairness and accuracy of AI systems. This emphasis on diversity in data labeling is becoming increasingly important as organizations recognize the potential risks of biased AI outputs, which can lead to unfair practices and legal challenges.
The market is also benefiting from advancements in automated and semi-automated data labeling technologies, which streamline the process and improve efficiency. However, human oversight remains essential to ensure the contextual accuracy and nuance required for high-quality AI training data. As AI models continue to evolve, the need for sophisticated data labeling solutions that balance automation with human expertise will become even more critical.
The generative AI in data labeling solution and services market is set for robust growth, driven by the increasing demand for high-quality, diverse, and relevant datasets. Companies that focus on innovation in data labeling technologies, while maintaining a strong commitment to reducing bias, will be well-positioned to lead in this dynamic and expanding market.
Key Takeaways
- By Sourcing Type: In-House data labeling leads with 55%, providing greater control over data quality and security.
- By Type: Image/Video-Based labeling constitutes 40%, essential for training AI models in computer vision.
- By Labeling Type: Semi-Supervised labeling represents 35%, balancing efficiency and accuracy in data annotation.
- By Vertical: IT Data accounts for 30%, reflecting the demand for accurate data labeling in technology-driven sectors.
- Regional Dominance: North America holds a 38% market share, driven by the high demand for AI and machine learning applications.
- Growth Opportunity: Integrating AI-driven tools to enhance the efficiency and accuracy of data labeling processes can significantly expand market opportunities.
Driving factors
Rising Demand for High-Quality Labeled Data as a Market Driver
The growth of the generative AI in data labeling solution and services market is significantly driven by the increasing demand for high-quality labeled data. In the development of AI and machine learning models, the quality of the input data directly impacts the performance and accuracy of these models. High-quality labeled data is essential for training AI systems to make precise predictions and decisions.
As businesses across various industries—such as healthcare, finance, and retail—continue to integrate AI into their operations, the demand for accurately labeled data has surged. This growing need for refined data labeling services fuels the expansion of the market, as organizations seek reliable solutions to meet their data needs.
Expansion of AI and Machine Learning Applications Boosts Market Growth
The proliferation of AI and machine learning applications across industries is another key factor driving the growth of the generative AI in data labeling solution and services market. As AI becomes integral to more business functions—ranging from predictive analytics to autonomous systems—the need for vast amounts of labeled data increases.
AI applications in areas such as natural language processing, computer vision, and speech recognition require large datasets that are meticulously labeled to train models effectively. This widespread adoption of AI amplifies the demand for data labeling services, positioning the market for sustained growth.
Advancements in AI for Automated Data Labeling Enhance Efficiency
Advancements in AI technologies, particularly in the realm of automated data labeling, are transforming the landscape of the data labeling market. Generative AI models have made significant strides in automating the labeling process, reducing the time and cost associated with manual data labeling. These advancements enable the rapid processing of large datasets, improving efficiency and scalability for businesses.
As automated data labeling technologies continue to evolve, they are expected to drive further growth in the market by providing more cost-effective and accurate labeling solutions, thereby meeting the rising demand for labeled data across various AI applications.
Restraining Factors
Data Accuracy and Quality Concerns as Significant Market Restraints
Despite the promising growth of the generative AI in data labeling solution and services market, concerns over data accuracy and quality present notable challenges. While generative AI models have made strides in automating the data labeling process, the accuracy of these labels remains a critical issue. Inaccurate labeling can lead to poorly trained AI models, which, in turn, can produce unreliable or biased outcomes.
This risk is particularly pronounced in industries where precision is paramount, such as healthcare and finance. As a result, companies may hesitate to fully embrace AI-driven labeling solutions, preferring traditional manual methods to ensure higher accuracy. These concerns can slow the adoption of generative AI in data labeling, constraining market growth.
High Costs of AI-Driven Labeling Solutions as a Barrier to Entry
The high costs associated with AI-driven data labeling solutions also act as a significant restraint on market growth. Developing and deploying generative AI models for data labeling requires substantial investment in technology, infrastructure, and skilled personnel. These costs can be prohibitive, especially for small and medium-sized enterprises (SMEs) that may lack the financial resources to implement such advanced solutions.
The ongoing expenses related to maintaining and updating AI-driven labeling systems add to the financial burden. As a result, the high cost of AI-driven labeling solutions may deter potential users, limiting market expansion and reducing the overall pace of adoption.
By Sourcing Type Analysis
In-house sourcing leads the generative AI data labeling market with a 55% share.
In 2023, In-House held a dominant market position in the "By Sourcing Type" segment of the Generative AI in Data Labeling Solution and Services Market, capturing more than a 55% share. The preference for in-house data labeling stems from the growing need for maintaining control over data quality and ensuring compliance with industry-specific regulations. Companies, particularly in highly regulated sectors like healthcare and finance, are increasingly opting for in-house solutions to safeguard sensitive information and customize labeling processes according to their unique requirements. The in-house approach also allows organizations to leverage internal expertise, ensuring that the labeled data aligns closely with their AI model objectives.On the other hand, the Outsourced segment, while essential for scalability and cost-effectiveness, accounted for a smaller share of the market. Outsourcing is often chosen by companies looking to handle large volumes of data quickly and cost-effectively, especially when internal resources are limited. Despite its advantages, concerns over data security, quality control, and the potential for communication gaps have led many organizations to favor in-house solutions, solidifying the segment's dominance.
By Type Analysis
Image and video-based labeling types dominate the market, holding a 40% share.
In 2023, Image/Video-Based held a dominant market position in the "By Type" segment of the Generative AI in Data Labeling Solution and Services Market, capturing more than a 40% share. The extensive use of image and video data in industries such as automotive, healthcare, and retail has driven the demand for precise and efficient data labeling services in this category. Generative AI technologies have significantly enhanced the ability to label large datasets of images and videos, enabling better training for AI models used in applications like autonomous vehicles, medical imaging, and retail analytics. The rise of computer vision applications has further bolstered the need for image and video-based data labeling.Audio-Based and Text-Based data labeling also played crucial roles in the market but captured smaller shares. Audio-Based labeling, essential for voice recognition and speech analysis applications, is gaining traction as voice-controlled technologies become more prevalent. Text-Based labeling, critical for natural language processing (NLP) and sentiment analysis, continues to be a significant segment, particularly in customer service and financial services. However, the complexity and volume of image and video data have led to their dominant position in the data labeling market.
By Labeling Type Analysis
Semi-supervised labeling methods account for 35% of the market.
In 2023, Semi-Supervised held a dominant market position in the "By Labeling Type" segment of the Generative AI in Data Labeling Solution and Services Market, capturing more than a 35% share. Semi-supervised labeling, which combines a small amount of labeled data with a larger set of unlabeled data, has gained popularity due to its efficiency and cost-effectiveness. This approach allows for the training of AI models with less manual intervention, reducing the time and resources required for data labeling while still achieving high accuracy. The growing complexity of AI models and the increasing volume of data have made semi-supervised labeling an attractive option for many organizations.The Manual and Automatic labeling segments, while still important, represented smaller portions of the market. Manual labeling, which offers the highest level of accuracy and control, is often used in highly specialized or critical applications but is limited by its scalability and cost. Automatic labeling, driven by AI and machine learning algorithms, is gaining momentum but is still constrained by its need for high-quality training data and the risk of inaccuracies. Despite these advances, the flexibility and efficiency of semi-supervised labeling have solidified its leading position in the market.
By Vertical Analysis
The IT data vertical dominates the market, representing 30% of the share.
In 2023, IT Data held a dominant market position in the "By Vertical" segment of the Generative AI in Data Labeling Solution and Services Market, capturing more than a 30% share. The IT sector's extensive use of AI and machine learning for various applications, including cybersecurity, predictive analytics, and software development, has driven the demand for robust data labeling solutions. The complexity and volume of data generated in the IT industry necessitate accurate and scalable labeling processes, making it the leading vertical in this market segment.Other verticals, such as Healthcare, Automotive, and Financial Services, also contributed significantly to the market. Healthcare, driven by the need for precise medical imaging and diagnostics, has seen a rise in the adoption of data labeling solutions. The Automotive sector, with its focus on autonomous driving and advanced driver-assistance systems (ADAS), relies heavily on labeled data for training AI models. Financial Services utilize data labeling for fraud detection and customer sentiment analysis, while Retail leverages it for personalized marketing and inventory management. Although these sectors are growing rapidly, IT Data remains the dominant force in the market due to its expansive and critical use of AI technologies.
Key Market Segments
By Sourcing Type
- In-House
- Outsourced
By Type
- Audio-Based
- Image/Video-Based
- Text-Based
By Labeling Type
- Automatic
- Manual
- Semi-Supervised
By Vertical
- Automotive
- Financial Services
- Government
- Healthcare
- IT Data
- Retail
- Others
Growth Opportunity
AI-Assisted Data Labeling Platforms Drive Market Innovation
In 2024, one of the most promising opportunities in the generative AI in data labeling solution and services market is the development and adoption of AI-assisted data labeling platforms. These platforms combine the efficiency of AI with human oversight, ensuring that the data labeling process is both accurate and scalable.
By leveraging AI to handle the more routine aspects of data labeling, these platforms allow human labelers to focus on complex and ambiguous cases, significantly improving the overall quality of the labeled data. This hybrid approach not only enhances the accuracy of AI models but also reduces the time and cost associated with data labeling, making it an attractive option for businesses looking to scale their AI operations.
Expansion in Autonomous Vehicles, Healthcare, and Finance Boosts Demand
The expansion of AI applications in key industries such as autonomous vehicles, healthcare, and finance presents a substantial growth opportunity for the generative AI in data labeling market. Autonomous vehicles, for instance, require vast amounts of labeled data to train models for object detection, navigation, and decision-making.
In healthcare, labeled medical data is crucial for developing AI models that assist in diagnostics and treatment planning. In the finance sector, AI-driven models rely on labeled data for tasks such as fraud detection and risk assessment. As these industries continue to grow and evolve, the demand for high-quality labeled data will rise, driving the need for advanced generative AI labeling solutions.
Latest Trends
Integration of Active Learning Enhances Data Labeling Efficiency
In 2024, the integration of active learning techniques into data labeling processes is emerging as a key trend in the generative AI market. Active learning allows AI systems to intelligently select the most informative data points that need labeling, thereby reducing the overall volume of data that requires manual annotation. This approach significantly enhances the efficiency and cost-effectiveness of data labeling, as it focuses human efforts on the most challenging and ambiguous cases.
By leveraging active learning, companies can improve the quality of their labeled datasets while also accelerating the training of AI models. This trend is expected to gain traction as organizations seek to optimize their data labeling workflows and reduce associated costs.
Use of AI for Synthetic Data Generation Expands Market Capabilities
Another significant trend in the 2024 generative AI in data labeling solution and services market is the increasing use of AI for synthetic data generation. Synthetic data, generated by AI algorithms, can be used to supplement real-world data, especially in scenarios where labeled data is scarce or difficult to obtain. This trend is particularly relevant in industries like autonomous vehicles, where generating labeled data for every possible scenario is impractical.
AI-driven synthetic data generation provides a solution by creating diverse and representative datasets that can be used to train models more effectively. As the technology for generating high-fidelity synthetic data improves, it is expected to play a crucial role in expanding the capabilities of the data labeling market.
Regional Analysis
North America leads the Generative AI in Data Labeling Solution and Services Market with a 38% share.
In 2023, North America held a dominant position in the Generative AI in Data Labeling Solution and Services Market, capturing 38% of the regional market share. This leadership is primarily driven by the region's advanced technological infrastructure, high adoption rates of AI, and the presence of key industry players. North America's dominance is further supported by significant investments in AI research and development, particularly in the United States, where major tech companies are leading the way in deploying generative AI for data labeling solutions.The region benefits from a robust ecosystem of startups, established enterprises, and research institutions that are continuously innovating in the field of AI. The demand for high-quality data labeling services is growing rapidly as organizations across various sectors, including automotive, healthcare, and finance, increasingly rely on AI-driven models that require vast amounts of accurately labeled data. This has led to the expansion of data labeling services, with a particular focus on generative AI techniques that enhance efficiency and accuracy.
North America's regulatory environment is conducive to AI innovation, with policies that encourage the development and deployment of AI technologies while ensuring data privacy and security. This has encouraged both domestic and international companies to invest in AI and related services in the region, further solidifying its market dominance.
Key Regions and Countries
North America
- US
- Canada
- Mexico
Western Europe
- Germany
- France
- The UK
- Spain
- Italy
- Portugal
- Ireland
- Austria
- Switzerland
- Benelux
- Nordic
- Rest of Western Europe
Eastern Europe
- Russia
- Poland
- The Czech Republic
- Greece
- Rest of Eastern Europe
APAC
- China
- Japan
- South Korea
- India
- Australia & New Zealand
- Indonesia
- Malaysia
- Philippines
- Singapore
- Thailand
- Vietnam
- Rest of APAC
Latin America
- Brazil
- Colombia
- Chile
- Argentina
- Costa Rica
- Rest of Latin America
Middle East & Africa
- Algeria
- Egypt
- Israel
- Kuwait
- Nigeria
- Saudi Arabia
- South Africa
- Turkey
- United Arab Emirates
- Rest of MEA
Key Players Analysis
The Generative AI in Data Labeling Solution and Services Market in 2024 is witnessing significant advancements, spearheaded by key players that are innovating to meet the growing demand for high-quality, scalable AI training data. Scale AI and DataRobot are leading the charge with their advanced data labeling platforms, which are increasingly being integrated into AI development pipelines across various industries. These companies are setting the standard for accuracy and efficiency in data labeling, crucial for training sophisticated AI models.
Amazon Web Services (AWS) and Google (DeepMind) are leveraging their cloud infrastructure and AI expertise to offer comprehensive data labeling solutions, enabling enterprises to scale their AI initiatives efficiently. IBM and Microsoft are also pivotal, focusing on integrating AI-driven data labeling into their broader AI and analytics ecosystems, thereby providing end-to-end solutions for data-driven decision-making.
Emerging players like Snorkel AI and iMerit are gaining traction with innovative approaches to data labeling, such as programmatic labeling and human-in-the-loop systems. These solutions are particularly attractive to organizations looking to reduce the time and cost associated with manual data labeling while maintaining high levels of accuracy.
Market Key Players
- Scale AI
- DataRobot
- Amazon Web Services (AWS)
- OpenAI
- Cognilytica
- Snorkel AI
- Google (DeepMind)
- iMerit
- IBM
- Slyce
- Playment
- CloudFactory
- Appen
- Trifacta
- Alegion
- Microsoft
- Labelbox
Recent Development
- In January 2024, Scale AI introduced an automated data labeling service powered by generative AI, increasing labeling efficiency by 40% and reducing human intervention.
- In March 2024, Labelbox secured $50 million in funding to enhance its AI-driven data labeling platform, aiming to improve data accuracy by 30% for its clients.
Report Scope
Report Features Description Market Value (2023) USD 11.9 Bn Forecast Revenue (2033) USD 84.0 Bn CAGR (2024-2033) 22.2% Base Year for Estimation 2023 Historic Period 2018-2023 Forecast Period 2024-2033 Report Coverage Revenue Forecast, Market Dynamics, Competitive Landscape, Recent Developments Segments Covered By Sourcing Type (In-House, Outsourced), By Type (Audio-Based, Image/Video-Based, Text-Based), By Labeling Type (Automatic, Manual, Semi-Supervised), By Vertical (Automotive, Financial Services, Government, Healthcare, IT Data, Retail, Others) Regional Analysis North America - The US, Canada, & Mexico; Western Europe - Germany, France, The UK, Spain, Italy, Portugal, Ireland, Austria, Switzerland, Benelux, Nordic, & Rest of Western Europe; Eastern Europe - Russia, Poland, The Czech Republic, Greece, & Rest of Eastern Europe; APAC - China, Japan, South Korea, India, Australia & New Zealand, Indonesia, Malaysia, Philippines, Singapore, Thailand, Vietnam, & Rest of APAC; Latin America - Brazil, Colombia, Chile, Argentina, Costa Rica, & Rest of Latin America; Middle East & Africa - Algeria, Egypt, Israel, Kuwait, Nigeria, Saudi Arabia, South Africa, Turkey, United Arab Emirates, & Rest of MEA Competitive Landscape Scale AI, DataRobot, Amazon Web Services (AWS), OpenAI, Cognilytica, Snorkel AI, Google (DeepMind), iMerit, IBM, Slyce, Playment, CloudFactory, Appen, Trifacta, Alegion, Microsoft, Labelbox Customization Scope Customization for segments, region/country-level will be provided. Moreover, additional customization can be done based on the requirements. Purchase Options We have three licenses to opt for: Single User License, Multi-User License (Up to 5 Users), Corporate Use License (Unlimited User and Printable PDF) -
-
- Scale AI
- DataRobot
- Amazon Web Services (AWS)
- OpenAI
- Cognilytica
- Snorkel AI
- Google (DeepMind)
- iMerit
- IBM
- Slyce
- Playment
- CloudFactory
- Appen
- Trifacta
- Alegion
- Microsoft
- Labelbox